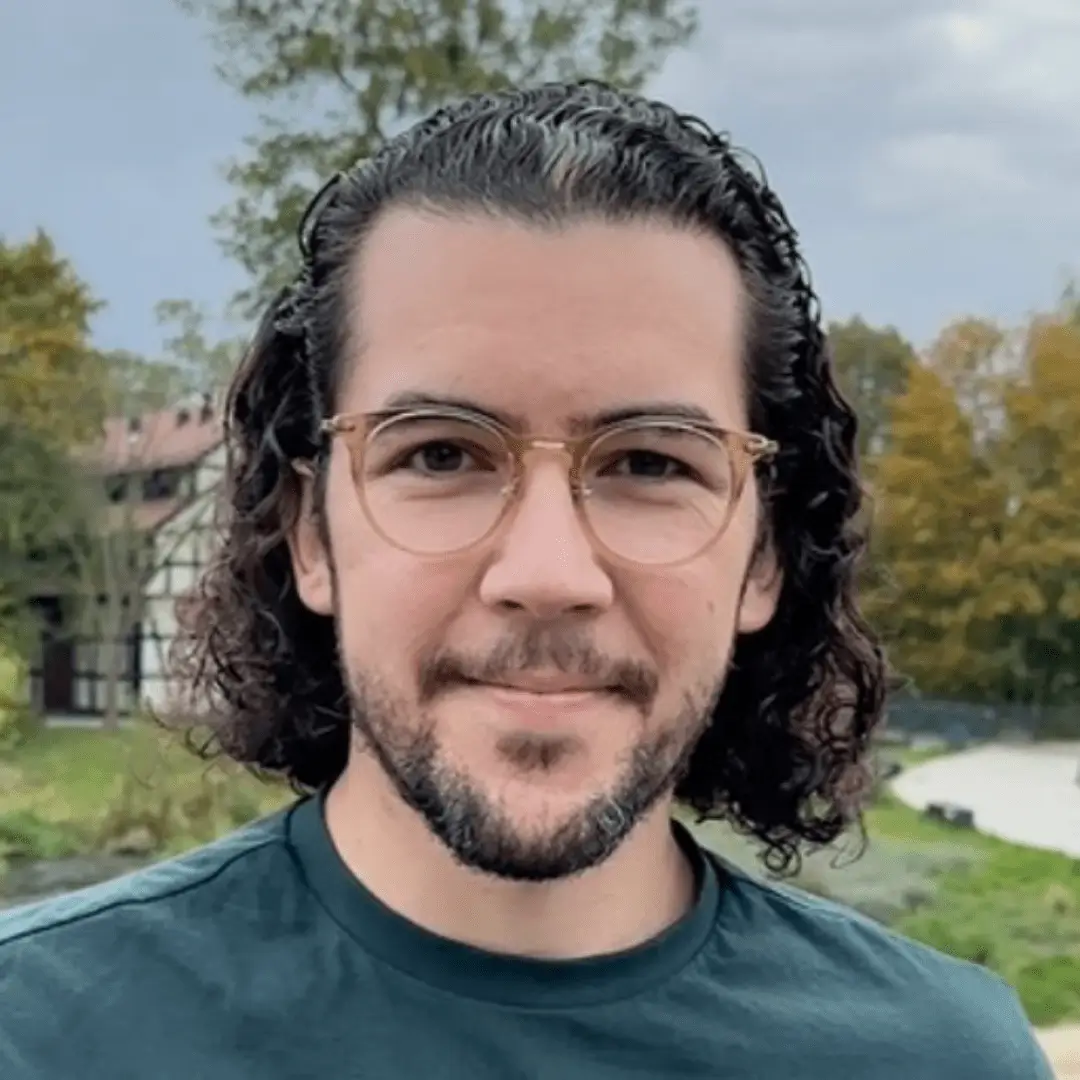
Our shared need for precision, fair play, and heightened tension during matches shapes a willingness to incorporate new sports technologies.
In a world where speed, efficiency, and customer experience are the gold standard, businesses are constantly looking for that magic bullet to streamline operations, boost productivity, and wow their customers. This is where conversational AI comes into play, a transformative technology that redefines organisations’ interactions with clients and optimises their internal processes.
At its core, conversational AI encompasses technologies like chatbots, virtual assistants, and advanced AI models, such as ChatGPT, designed to simulate human-like conversations. But gone are those days when chatbots were just managing simple queries. Modern AI models, particularly large language models (LLMs), are capable of much more. They are smarter, faster, and can help companies optimise workflows, make data-driven decisions, and, yes, actually increase revenue. And here’s the kicker: they’re doing all of this without needing a day off.
The importance of conversational AI cannot be overstated. According to a report by Grand View Research, the global conversational AI market size was valued at USD 4.91 billion in 2020 and is expected to expand at a compound annual growth rate (CAGR) of 21.8% from 2021 to 2028.
But let’s not get ahead of ourselves. As companies seek to leverage these tools, the question arises: how can conversational AI be effectively integrated into business strategies? And that’s exactly what we’re here to explore.
In this article, we’ll take a close look at the benefits of conversational AI for businesses, explain how these models work, and even present some real-world case studies to illustrate this tech profound impact. Ready? Let’s uncover the potential of conversational AI and learn how to make it a game-changer for your business.
The rise of conversational AI is a strategic advantage that businesses can no longer afford to overlook. It has become a vital component in optimising business operations, offering organisations significant opportunities to streamline internal processes. By leveraging advanced AI models, businesses can automate repetitive tasks, boost productivity, and ensure operational efficiency without breaking a sweat.
AI models, such as chatbots and virtual assistants, can manage a variety of administrative functions – from scheduling meetings to addressing customer inquiries and processing transactions. This automation not only saves valuable time but also cuts down the risk of human error, ensuring your operations run smoother than a well-oiled machine.
Conversational AI can handle the tasks that typically eat up hours of employee time. Think of it as the perfect assistant who doesn’t take breaks, doesn’t get tired, and can handle customer service inquiries, scheduling, order tracking, or even internal HR requests 24/7. By integrating AI into workflows, companies can drastically reduce the time spent on low-value activities, freeing up teams to focus on strategic projects.
For example, AI chatbots in a customer support team can respond instantly to common queries, reducing the need for human intervention in 60-70% of cases. And for internal processes, AI can handle routine employee requests, such as booking leave or checking payroll status, instantly.
For example, a report by Gartner suggests that by 2022, 70% of customer interactions will involve emerging technologies such as machine learning applications, chatbots, and mobile messaging. Additionally, IBM has reported that chatbots can handle up to 80% of routine customer service interactions.
Need a hand with your custom chatbot development? Contact us!
Conversational AI doesn’t just perform tasks – it learns. Over time, these systems analyse patterns and suggest better ways to allocate resources, whether it’s staff, budget, or time.
AI-driven insights can help managers make informed decisions about where to deploy teams or optimise processes that need more attention. This leads to more effective operations and less time wasted on inefficient workflows.
By integrating conversational AI with existing systems like CRM or ERP, businesses can get real-time updates and access to key performance metrics without manual reporting. This makes decision-making quicker and more data-driven. Imagine a sales team where managers no longer have to sift through reports to get a sense of team performance – AI-driven assistants can deliver the data instantly.
Conversational AI models like ChatGPT are built to process and analyse large datasets, delivering valuable insights that inform strategic decisions. These systems can pull meaningful information from both structured and unstructured data sources – think customer feedback, market trends, and operational metrics.
By interpreting this data, AI models can provide recommendations that align perfectly with your business goals and market demands.
AI-driven systems can handle a wide range of tasks, from answering frequently asked questions to processing transactions and providing technical support. Here are some key benefits:
If you still have doubts, let the numbers speak – here are some statistics to show how conversational AI improve customer interactions:
For businesses looking to explore the integration of AI into their operations, we also recommend getting acquainted with our article on the software development process, which is crucial to ensure successful implementation.
Conversational AI can reformat and personalise text on the fly. For businesses managing diverse customer bases – ranging from tech-savvy startups to traditional enterprise clients – this adaptability is crucial. Instead of offering a one-size-fits-all answer, AI can rework the tone, complexity, and structure of responses depending on the recipient’s needs. Whether you need a friendly tone for casual inquiries or a more formal approach for high-stakes clients, AI can shift gears effortlessly.
By using natural language processing (NLP), AI rephrases text without losing the core message, delivering consistency across channels while maintaining the flexibility to suit individual preferences. Imagine a customer asking about a product feature – conversational AI can provide a high-level overview for casual users while diving deep into technical specs for a more expert audience.
A well-organised knowledge base is the backbone of efficient customer service and employee training. AI doesn’t just automate responses – it helps structure and personalise knowledge repositories to make them more accessible and useful. By using conversational AI, businesses can customise and update their knowledge bases in real-time, ensuring that the most relevant, up-to-date information is always available.
For example, AI can pull from multiple sources: technical manuals, FAQs, or even past customer interactions to offer the most contextually appropriate answer. Additionally, businesses can set custom parameters to prioritise certain types of information or tailor responses based on customer profiles, location, or previous engagements. This level of customisation enhances the user experience and reduces the need for costly human intervention.
In fast-moving industries, keeping knowledge bases up to date can be a challenge. With conversational AI, businesses can automatically sync updates from new product releases, service changes, or regulatory updates into their knowledge bases. This ensures that AI-driven interactions reflect the latest information, avoiding outdated or incorrect responses. Whether it’s legal requirements, product specifications, or policy changes, AI adapts in real-time, so your knowledge base always stays relevant.
Conversational AI has moved beyond traditional applications such as customer service and virtual assistants. Its versatility is being harnessed in various innovative and unconventional ways across different sectors. These non-standard uses are expanding the horizons of what AI can achieve.
Several sectors are pioneering the use of conversational AI in unexpected ways:
For years, businesses have relied on custom, rule-based bots to handle specific tasks – automating basic customer service inquiries, directing users to certain sections of websites, or processing simple requests. While these bots served their purpose, the increasing complexity of modern business needs has revealed their limitations. Enter conversational AI models like ChatGPT, which are pushing the boundaries of what’s possible in terms of flexibility, understanding, and contextual interaction.
Custom bots, or rule-based bots, operate by following a pre-defined script or set of rules. They’re good at handling repetitive, structured tasks – like answering FAQs or guiding users through fixed processes. But the moment a request falls outside of their programmed responses, they hit a wall. These bots struggle with anything that requires nuance, understanding of context, or flexibility in responses.
For example, a typical rule-based bot might handle an inquiry about business hours but falter when asked a more complex, multi-layered question, such as comparing product features based on customer needs. This lack of adaptability makes them ill-suited for dynamic industries that require more advanced interactions.
As customer interactions grow more sophisticated, businesses need tools that can evolve alongside them. Rule-based bots simply can’t keep up with the demands of today’s users. They can’t handle complex queries, don’t learn from interactions, and tend to offer generic solutions. This often leads to frustration, with customers feeling like they’re speaking to a machine – because, well, they are.
For businesses, this rigidity can cause operational inefficiencies, with human agents having to step in whenever a bot gets stuck. Moreover, with customer expectations at an all-time high, providing a seamless, intelligent interaction is crucial for maintaining competitive advantage.
Conversational AI models, on the other hand, are designed to be more than just reactive tools – they’re proactive problem-solvers. Unlike traditional bots, they don’t rely solely on scripts. They learn from each interaction and can adapt to a wide range of inputs, even those that are more ambiguous or open-ended.
Natural Language Understanding (NLU): Conversational AI understands and processes natural language. This allows it to engage in more human-like conversations, grasping nuances, detecting sentiment, and responding accordingly.
Context Awareness: Unlike rule-based bots, conversational AI remembers context within a conversation. If a customer asks about product details, and then later inquires about pricing, the AI can retain the context of the original product, making the interaction feel smoother and more personalised.
Continuous Learning: These models learn and improve over time. With each interaction, conversational AI refines its responses, gaining insights into how to handle more complex queries in the future.
For example, while a custom bot might offer a one-size-fits-all response to a service inquiry, conversational AI can offer personalised responses based on previous interactions, past purchases, or user preferences. It can even provide proactive recommendations, offering solutions before a customer realises they need them.
In short, while traditional custom bots once provided sufficient support for basic tasks, they can’t match the adaptability, depth, or intelligence of conversational AI models. As businesses face increasingly complex demands, upgrading to AI-driven models ensures a more flexible, insightful, and human-like interaction that meets the needs of today’s markets.
While conversational AI offers numerous benefits, its integration into business operations is not without risks and challenges. Some businesses even prohibit the use of AI chat due to ethical concerns. So, understanding these potential pitfalls is crucial for businesses aiming to leverage AI technologies effectively.
Want to implement AI solutions in a secure and safe way? We’re here to help. Let’s talk!
Data Privacy and Security Threats: AI systems often handle sensitive data, raising concerns about unauthorised access, data breaches, and non-compliance with regulations like GDPR. This is especially critical in industries like healthcare and finance.
Inaccurate Responses: AI models can produce incorrect or biased answers, especially with complex queries, which may lead to misinformation in sectors like law, healthcare, or finance.
Bias and Fairness: AI systems can inherit biases from training data, leading to discriminatory outcomes. Ensuring fairness and reducing bias requires continuous monitoring.
Job Displacement: The automation of tasks through AI may lead to job losses, raising ethical concerns about the balance between technology and human employment.
Legal and Regulatory Challenges: Unclear legal responsibilities and evolving regulations around AI usage make businesses hesitant to fully adopt AI technologies.
Technical Complexity: Developing and deploying AI systems require specialised expertise and significant resources. Many businesses may struggle with the technical complexity of AI integration. Or costs can be quite high, so optimisation is a necessity – contact us and our team will be glad to to assist with that process.
Change Management: Implementing AI solutions often necessitates changes in existing workflows and processes. Ensuring that employees are trained and prepared for these changes can be a significant challenge.
Cost: The initial investment for AI implementation can be substantial, encompassing costs for development, deployment, and ongoing maintenance.
Ethical Considerations: Businesses must navigate the ethical implications of AI, including ensuring transparency, accountability, and responsible use of AI technologies.
Businesses can adopt several best practices to mitigate the risks associated with conversational AI:
Data Encryption: Implement robust data encryption methods to protect sensitive customer information and ensure compliance with data privacy regulations.
Regular Audits: Conduct regular audits and assessments of AI systems to identify and rectify biases, ensuring that the AI operates fairly and ethically.
Redundancy Systems: Develop redundancy systems and backup plans to mitigate the impact of technical failures or downtimes, ensuring business continuity.
Employee Training: Provide comprehensive training programs for employees to familiarise them with AI technologies and prepare them for changes in workflows and processes.
Cybersecurity Measures: Implement advanced cybersecurity measures to protect AI systems from cyber-attacks and ensure the integrity and security of AI operations.
By understanding and proactively addressing the risks and challenges of integrating conversational AI, businesses can harness the transformative potential of AI technologies while safeguarding their operations and customer trust.
As conversational AI continues to evolve, businesses are faced with a range of AI models to choose from – each with its own strengths. Let’s break down a few of the leading AI models and see how they stack up in terms of performance, cost, and versatility.
Model
Capabilities
Pricing
Use Cases
ChatGPT
(OpenAI)
Strong general-purpose text generation, customizable, wide integration
GPT-3.5: $0.0015 - $0.002 / 1k tokens. GPT-4: $0.03+ / 1k tokens
Versatile, general applications, enterprise use
Gemini
(Google)
Strong integration with Google services, customizable via Vertex AI
Pay-as-you-go via Google Cloud; specifics vary with usage
Enterprise applications, Al-powered services
Claude
(Anthropic)
Safe, reliable, less prone to harmful content, focused on ethics
Per-call pricing; specifics depend on enterprise contracts
Highly ethical environments, chatbot, content review
LLaMA
(Meta)
Open-source, fine-tuning possible, high flexibility
Free for model weights, but compute costs for deployment
Research, open-source projects, self-hosted AI
Source: Own research
LLaMA is well-suited for developers and researchers who want to build highly customised AI systems, with more control over the model’s parameters. Its open-source nature gives it a flexibility that’s unmatched in some areas but lacks the user-friendly ecosystem and seamless business integrations seen in other solutions.
Claude is a strong choice for organisations that prioritise AI safety and ethics, particularly in sensitive areas like customer service and moderation. It’s not the most flexible or creative option, but it offers strong alignment principles that ensure its responses are reliable and safe.
Gemini is designed for advanced conversational use cases, particularly where reasoning, multi-step problem solving, and planning are critical. Its integration with Google’s ecosystem makes it a great choice for enterprise customers who need cutting-edge AI solutions with access to vast data sets and computational resources.
ChatGPT remains a versatile and highly capable general-purpose model, excelling in content generation, coding, creative writing, and knowledge tasks. However, it lacks the deep focus on safety seen in Claude or the advanced planning skills of Gemini.
Need support with building your own conversational solution? Contact us
Selecting the right conversational AI solution requires a careful evaluation of your business needs, budget, integration complexity, and long-term goals. Let’s break down the key factors to consider when choosing a conversational AI model, and explore the associated costs, complexity, and performance:
Industry and Use Case: The nature of your business and its core needs play a crucial role in selecting a conversational AI solution. For instance:
As your business grows, your conversational AI solution must be able to handle an increasing number of interactions and queries.
Evaluating the pricing models is crucial, especially for businesses with budget constraints. Conversational AI solutions generally charge based on API calls, tokens (word counts), or subscription tiers. Below is a general idea of cost structures:
Assess the ease of integration with your existing systems, whether it’s a CRM, website, or other internal tools:
Response time is critical, especially for customer-facing applications. AI platforms hosted on major cloud infrastructures (e.g., AWS, Google Cloud, Microsoft Azure) generally offer low-latency responses. Some considerations:
Data security and user privacy are major concerns, particularly for industries like healthcare and finance:
For smaller businesses, ChatGPT is an excellent starting point due to its low-cost entry, ease of use, and general-purpose versatility. If you’re embedded in the Google ecosystem, Dialogflow or Gemini may be ideal for scaling customer interactions or leveraging complex reasoning tasks. Enterprise organisations with more specific demands around privacy, security, and scalability often turn to IBM Watson Assistant or Claude, which prioritise ethical, secure, and reliable interactions.
By thoroughly assessing these factors, businesses can make informed decisions and select a conversational AI solution that best meets their operational goals and budget constraints.
If you are unsure or need individual advice, contact us and we will help you determine the best solution based on your unique requirements.
As businesses continue to navigate the complexities of the modern landscape, the integration of conversational AI offers a compelling opportunity for innovation. The ability to dynamically rework text and customise knowledge bases enables organisations to communicate more effectively, adapting to the unique needs of their customers and internal teams.
By leveraging conversational AI, businesses can move beyond traditional methods, fostering a more agile and responsive operational environment. This technology not only enhances efficiency but also enriches the customer experience, creating interactions that feel more personal and relevant.
Looking ahead, organisations that embrace these advanced AI capabilities will likely gain a competitive edge, unlocking new avenues for growth and engagement. The path forward is clear: conversational AI is not just a tool for efficiency; it’s a catalyst for transformation, paving the way for smarter, more connected business practices.